How to Spot and Destroy Evil Attractors in Your Tree (Part 1)
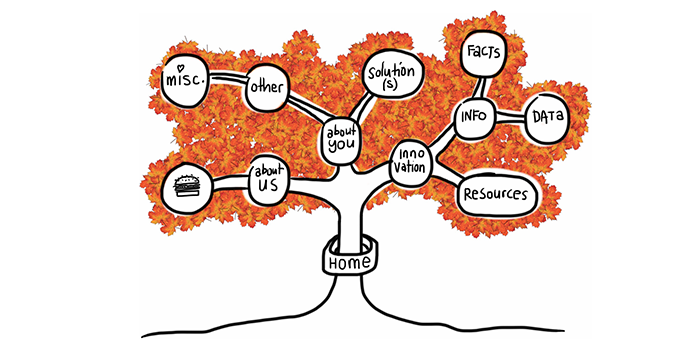
Usability guru Jared Spool has written extensively about the ‘scent of information’. This term describes how users are always ‘on the hunt’ through a site, click by click, to find the content they’re looking for. Tree testing helps you deliver a strong scent by improving organisation (how you group your headings and subheadings) and labelling (what you call each of them).
Anyone who’s seen a spy film knows there are always false scents and red herrings to lead the hero astray. And anyone who’s run a few tree tests has probably seen the same thing — headings and labels that lure participants to the wrong answer. We call these ‘evil attractors’.
In Part 1 of this article, we’ll look at what evil attractors are, how to spot them at the answer end of your tree, and how to fix them. In Part 2, we’ll look at how to spot them in the higher levels of your tree.
The false scent — what it looks like in practice
One of my favourite examples of an evil attractor comes from a tree test we ran for consumer.org.nz, a New Zealand consumer-review website (similar to Consumer Reports in the USA). Their site listed a wide range of consumer products in a tree several levels deep, and they wanted to try out a few ideas to make things easier to find as the site grew bigger.
We ran the tests and got some useful answers, but we also noticed there was one particular subheading (Home > Appliances > Personal) that got clicks from participants looking for very different things — mobile phones, vacuum cleaners, home-theatre systems, and so on:
The website intended the Personal appliance category to be for products like electric shavers and curling irons. But apparently, Personal meant many things to our participants: they also went there for ‘personal’ items like mobile phones and cordless drills that actually lived somewhere else.
This is the false scent — the heading that attracts clicks when it shouldn’t, leading participants astray. Hence this definition: an evil attractor is a heading that draws unwanted traffic across several unrelated tasks.
Evil attractors lead your users astray
Attracting clicks isn’t a bad thing in itself. After all, that’s what a good heading does — it attracts clicks for the content it contains (and discourages clicks for everything else).
Evil attractors, on the other hand, attract clicks for things they shouldn’t. These attractors lure users down the wrong path, and when users find themselves in the wrong place they’ll either back up and try elsewhere (if they’re patient) or give up (if they’re not). Because these attractor topics are magnets for the user’s attention, they make it less likely that your user will get to the place you intended.
The other evil part of these attractors is the way they hide in the shadows. Most of the time, they don’t get the lion’s share of traffic for a given task. Instead, they’ll poach 5–10% of the responses, luring away a fraction of users who might otherwise have found the right answer.
Find evil attractors easily in your data
The easiest attractors to spot are those at the answer end of your tree (where participants ended up for each task). If we can look across tasks for similar wrong answers, then we can see which of these might be evil attractors.
In your Treejack results, the Destinations tab lets you do just that. Here’s more of the consumer.org.nz example:
Normally, when you look at this view, you’re looking down a column for big hits and misses for a specific task. To look for evil attractors, however, you’re looking for patterns across rows. In other words, you’re looking horizontally, not vertically.
If we do that here, we immediately notice the row for Personal (highlighted yellow). See all those hits along the row? Those hits indicate an attractor — steady traffic across many tasks that seem to have little in common.
But remember, traffic alone is not enough. We’re looking for unwanted traffic across unrelated tasks. Do we see that here?
Well, it looks like the tasks (about cameras, drills, laptops, vacuums, and so on) are not that closely related. We wouldn’t expect users to go to the same topic for each of these. And the answer they chose, Personal, certainly doesn’t seem to be the destination we intended. While we could rationalise why they chose this answer, it is definitely unwanted from an IA perspective.
So yes, in this case, we seem to have caught an evil attractor red-handed. Here’s a heading that’s getting steady traffic where it shouldn’t.
Evil attractors are usually the result of ambiguity
It’s usually quite simple to figure out why an item in your tree is an evil attractor. In almost all cases, it’s because the item is vague or ambiguous — a word or phrase that could mean different things to different people.
Look at our example above. In the context of a consumer-review site, Personal is too general to be a good heading. It could mean products you wear, or carry, or use in the bathroom, or a number of things. So, when those participants come along clutching a task, and they see Personal, a few of them think ‘That looks like it might be what I’m looking for’, and they go that way.
Individually, those choices may be defensible, but as an information architect, are you really going to group mobile phones with vacuum cleaners? The ‘personal’ link between them is tenuous at best.
Destroy evil attractors by being specific
Just as it’s easy to see why most attractors attract, it’s usually easy to fix them. Evil attractors trade in vagueness and ambiguity, so the obvious remedy is to make those headings more concrete and specific.
In the consumer-site example, we looked at the actual content under the Personal heading. It turned out to be items like shavers, curling irons, and hair dryers. A quick discussion yielded Personal care as a promising replacement — one that should deter people looking for mobile phones and jewellery and the like.
In the second round of tree testing, among the other changes we made to the tree, we replaced Personal with Personal Care. A few days later, the results confirmed our thinking. Our former evil attractor was no longer luring participants away from the correct answers:
Testing once is good, testing twice is magic
This brings up a final point about tree testing (and about any kind of user testing, really): you need to iterate your testing — once is not enough.
The first round of testing shows you where your tree is doing well (yay!) and where it needs more work so you can make some thoughtful revisions. Be careful though. Even if the problems you found seem to have obvious solutions, you still need to make sure your revisions actually work for users, and don’t cause further problems.
The good news is, it’s dead easy to run a second test, because it’s just a small revision of the first. You already have the tasks and all the other bits worked out, so it’s just a matter of making a copy in Treejack, pasting in your revised tree, and hooking up the correct answers. In an hour or two, you’re ready to pilot it again (to err is human, remember) and send it off to a fresh batch of participants.
Two possible outcomes await.
- Your fixes are spot-on, the participants find the correct answers more frequently and easily, and your overall score climbs. You could have skipped this second test, but confirming that your changes worked is both good practice and a good feeling. It’s also something concrete to show your boss.
- Some of your fixes didn’t work, or (given the tangled nature of IA work) they worked for the problems you saw in Round 1, but now they’ve caused more problems of their own. Bad news, for sure. But better that you uncover them now in the design phase (when it takes a few days to revise and re-test) instead of further down the track when the IA has been signed off and changes become painful.
Stay tuned for more on evil attractors
In Part 1, we’ve covered what evil attractors are and how to spot them at the answer end of your tree: that is, evil attractors that participants chose as their destination when performing tasks. Hopefully, a future version of Treejack will be able to highlight these attractors to make your analysis that much easier.
In Part 2, we’ll look at how to spot evil attractors in the intermediate levels of your tree, where they lure participants into a section of the site that you didn’t intend. These are harder to spot, but we’ll see if we can ferret them out.
Let us know if you’ve caught any evil attractors red-handed in your projects.